Overview
Cluster randomized trials are trials in which clusters of individuals rather than individuals themselves are randomly allocated to different intervention groups. We then obtained hierarchical data with participants embedded in clusters. Cluster randomized trials may have clinical settings and thus randomize practices, health professionals, wards, hospitals, etc. or non-clinical settings and randomize schools, families, residential areas, worksites, etc.
These trials find applications in clinical research, but also in population health intervention research which often assesses complex interventions. Examples of complex interventions include the setting up of new healthcare teams, interventions to adopt treatment guidelines, whole community education interventions, etc. Randomizing clusters then avoids group contamination, which would occur due to interactions between members of a common cluster being allocated to different groups.
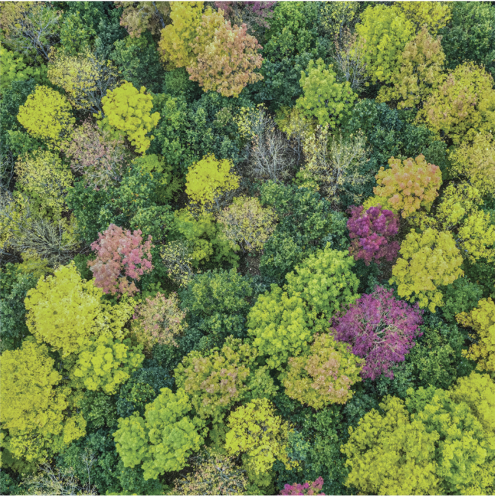
The cluster randomized design has methodological and statistical challenges.
Bias is notably an issue due to the fact that in most cluster randomized trials, clusters are recruited and randomized, and only then participants are recruited. Such a timeline, which differs from the usual timeline encountered in a classical individually randomized trial, may favor bias, a topic on which we worked in our unit :
1 - We showed that propensity score methods were of no interest to prevent bias in estimating an intervention effect in a cluster randomized trial subject to baseline imbalance
Read the publication Read the publication
2 - We developed a method based on a propensity score approach to identify baseline imbalance in cluster randomized trials
Read the publication
3 - We developed a graphical tool (the Timeline cluster) depicting the time sequence of steps and blinding status in cluster randomized trials
Read the publication
We also developed a website to help people in using our tool
Link to Timeline Cluster
Timeline Cluster Illustration
4 - We conducted a meta-epidemiological study comparing cluster randomized trials to individually randomized trials. We did not find any difference in intervention effect estimates between the two designs when the outcome is binary, while results were less clear for continuous outcomes
Read the publication
We also worked on issues raised specifically for binary data in cluster randomized trials such as the impact of dichotomizing a continuous outcome on power, how to handle missing binary data or how to assess clustering :
1 - We showed that dichotomizing a continuous outcome in the framework of a cluster randomized trial leads to a loss of power, but less important as compared to the context of an individually randomized trial. This is the consequence of a reduction of the intracluster correlation
Read the publication
2 - In a simulation study we compared different methods to cope with missing binary data in a cluster randomized trial and found that both multiple imputation with a random-effects logistic regression model or classical logistic regression are poorly biased methods and that both show good coverage properties
Read the publication
3 - Estimating intracluster correlation is challenging when the outcome is binary. The R coefficient has been suggested to be less dependent to the outcome prevalence than the intracluster correlation coefficient, but we showed that this is not true
Read the publication
Currently, we pursue our statistical developments notably considering time-to-event outcomes. Thus, the QUARTET project (ANR funded) aims at comparing statistical methods to be used for such a type of outcome. It also aims at defining a measure of clustering for such a kind of outcome. Other methodological works are also ongoing, notably the POPULAR project (Ministry of Health PREPS funding) which aims at better defining intention to treat population in a cluster randomized trial.
Most of these methodological and statistical works are generally conducted in collaboration with colleagues from the UK or Canada.
Pr Sandra Eldridge (UK) see her personal page,
Dr Monica Taljaard (Canada) see her personal page and
Pr Charles Weijer (Canada) see his personal page.
In parallel, we also aim at developing complex interventions and further assess their effect.
Complex interventions are generally developed using mixed research (quantitative and qualitative researches) as for instance in the ISAMA project (CHRU de Tours funded) which focuses on developing an intervention to detect and prevent Alzheimer patient caregiver overload (either physical, emotional or financial).
Such interventions are later assessed using a cluster randomized design, as for instance the EHPAGE project (Ministry of Health PREPS funding) which deals with the implementation of a coaching model (methodological help for professionals on the patient safety culture) to prevent adverse events in nursing homes.